Greetings from a world where…
ultimate frisbee could become an Olympic sport one day
…As always, the searchable archive of all past issues is here. Please please subscribe here to support ChinAI under a Guardian/Wikipedia-style tipping model (everyone gets the same content but those who can pay support access for all AND compensation for awesome ChinAI contributors).
Feature Translation: Behind the craze to build AI computing centers
Context: Last month, QbitAI (量子位) published a report on China’s effort to build computing centers to accelerate AI applications. Not many people voted for this article in the most recent Around the Horn, but I thought it was cool. Insert grandiose statement about the purpose of curators to show us what we really want as opposed to what we say we want. I say the people really want technical specifications about computing clusters!
Key Takeaways:
The goal: Let the computing power flow like tap water (让算力像自来水一样流淌)
AI computing centers as “essential infrastructure” in all parts of the country. As the article reports, Xi’an, Xuchang, Nanjing, Hangzhou, Guangzhou, Dalian, Qingdao, Changsha, Taiyuan, Nanning, are among the cities that have started building or are planning to build computing centers to support AI applications.
Four such computing centers have already been built. I think the PCL supercomputing center in Shenzhen (ChinAI #73) is one of them? ***Bonus points to the ChinAI reader that can track down the others.
The problems are twofold:
1) Price chaos — In one city, the construction cost for a computing center with performance of 100 PFlops (100P) at 16-bit precision is 75 million RMB. In another city, a computing center with the same specifications costs 450 million RMB, a difference of 6.2 times.
2) Confusion over how to benchmark compute clusters — different applications have varying requirements for precision. For instance, AI model training mainly uses 32-bit single-precision; AI inference (model implementation) can use 16-bit or lower. By contrast, some scientific calculations, such as weather forecasting or drug discovery, require higher 64-bit double precision. In the current rush to build computing centers, there’s been confusion over these different precision requirements. Specifically, the piece calls out the inflated prices for computing centers with high peak performance metrics (measured in PFlops) but low precision: these are deceptive gimmicks that “pass off fish eyes as pearls” [鱼目混珠] and can’t meet industrial needs.
The report warns, “If these two problems are not resolved, the smart computing centers built will not match the true value in price, nor can it meet the corresponding demand, which will inevitably cause waste of resources and hinder the development of the industry.”
What’s the potential solution?
The report emphasizes standardization and stable benchmarks, specifically higlighting efforts by the Chinese Academy of Sciences AI Industry-University-Research Innovation Alliance [中科院人工智能产学研创新联盟]. At the World AI Confernece 2021, this CAS alliance released a new generation AI computing platform, which aimed to set the standard for intelligent computing centers.
The key here is that many AI application scenarios, including material design and drug discovery, require a combination of AI and high-precision scientific computing. Toward that end, this platform “supports a multi-chip combination of CPUs, general-purpose GPUs, and dedicated AI acceleration chips, providing computing power covering various precisions, and can be competent for simulation, training, inference and other AI full-chain application requirements.
As for stabilizing prices, the CAS alliance gave out this guidance: “After integrating a series of factors such as storage, energy consumption, development, customization, and data scheduling, as well as plugging in clear algorithm standards, for an intelligent computing center with 5P double-precision computing power (64-bit), 25P single-precision computing power (32-bit), and 100P half-precision computing power (16 bits), the resulting infrastructure price is about 100 million-150 million RMB.”
Dig Deeper
Okay, I know we’re already in the weeds but let’s drill down even more and add some historical context. I think we can uncover a similar theme — impressive top-line numbers paired with underutilization — in China’s previous efforts to build supercomputers.
See this 2010 Science article on Dawning 5000A, which was once China’s fastest supercomputer: “Only 1% of the applications on China’s previous speed champ, the Dawning 5000A at the Shanghai Supercomputer Center, use more than 160 of the machine’s 30,720 cores. For comparison, 18% of the applications running on Oak Ridge’s Jaguar XT5 use 45,000 to 90,000 of the machine’s 150,162 cores, according to a presentation at last year’s announcement of China’s top 100 fastest computers. ‘A supercomputer without software is like a wild horse without a harness,’ says Zhang Yunquan, a parallel computing researcher at the Institute of Software of the Chinese Academy of Sciences in Beijing. ‘Its horsepower is wasted.’”
Brian Tsay, in a 2013 SITC Bulletin piece, writes, “it is not easy to write code that can actually utilize all the computing power that an HPC (high-performance computing) system has to offer. The result is that supercomputers can be left idle for long periods of time, raising the question of whether China even needs greater computing capacity.”
My favorite passage from Brian’s piece, which discusses China’s Tianhe-2 (TH-2), once the fastest supercomputer in the world: “For example, in response to the notion that the TH-2 will be used to improve China’s automobile industry, a professor at Tsinghua University’s department of automobile engineering commented, ‘I have never heard of Toyota or Daimler or any major carmaker using a supercomputer to design their cars [...] It is like running after a chicken with an axe. It is quite unnecessary.’”
***FULL TRANSLATION: Behind the craze to build AI computing power centers: who is spending pointless money
ChinAI Links (Four to Forward)
Must-read: Survey of Machine Learning Researchers on Ethics and Governance of Artificial Intelligence
Informative and surprising survey results published by a team of GovAI and UPenn researchers: Authors from NeurIPS and ICML (two top ML conferences) entrust international and scientific organizations to manage AI development; they place moderate trust in western tech companies and much lower trust in Chinese tech companies. One puzzle: when asked which AI governance challengers they were most worried about, researchers rated U.S.-China competition as the lowest of all risks. For more context, see this Twitter thread by Markus Anderljung, one of the co-authors.
Should-read: China Information Operations Newsletter
Really impressed by latest issue of the monthly China Information Operations Newsletter, edited by Hannah Bailey and Hannah Kirk, based at the Programme on Democracy and Technology at Oxford University. They not only digest the latest news on information operations but also place it in conversation with relevant academic journal articles and books.
Should-read: In US and China, Competition Rhetoric Meets Inequality Concerns
Every few months or so, I try to catch up on translations I’ve missed from various sources, including China Digital Times. This one caught my eye. Back in April 2021, John Chan wrote about this “rare glimpse at the sentiment of critical Chinese netizens”:
Chinese netizens made use of a rare window of opportunity to criticize China’s own inequality issues in response to their government’s foreign policy talk. The criticism was kicked off by a Xinhua interview with Chinese Foreign Minister Wang Yi that was published on Monday, in which Wang warned the U.S. against taking a “superior position” in world affairs…
Wang’s interview was disseminated widely across domestic Chinese media. But it received particular attention from Weibo netizens after it was reposted on Phoenix Television’s official account, when users discovered that their comments were not being intercepted by censors. The comments section was quickly overrun, after netizens took issue with Wang’s statement about “superiority,” which in Chinese could alternately be translated as “China doesn’t recognize that there is a country with ‘superior people’ in this world.”
Widely upvoted comments decried the hypocrisy of Wang’s remarks, as users pointed out China’s own problems with social inequality. Netizens latched onto Wang’s “superior people” remark to criticize China’s social services for privileging so-called “superior people” over ordinary citizens. Others protested that central Party leaders delivered “empty talk and bluster.” Thousands of comments were later deleted from the post, but not before CDT editors collected a selection (originally in Chinese):
Should-read: Security Studies Vol. 30, Issue 2

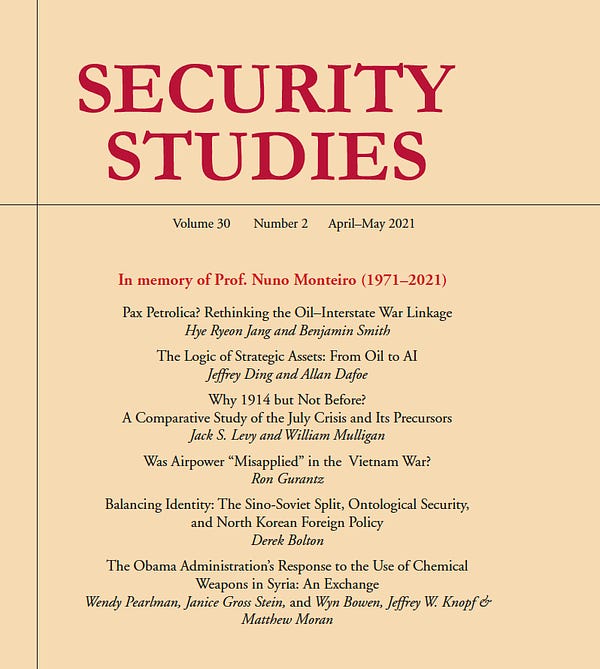
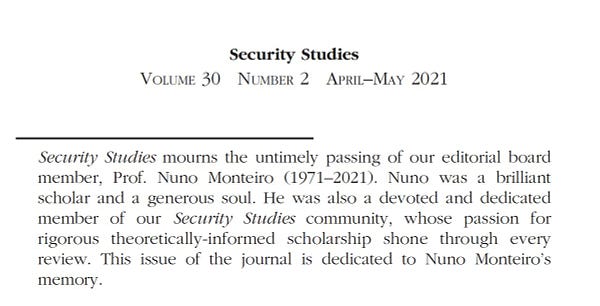
The latest issue of Security Studies, which includes my article (co-authored with Allan Dafoe) — “The Logic of Strategic Assets: From Oil to AI” — is now online. Check it out and let me know what you think!
Thank you for reading and engaging.
These are Jeff Ding's (sometimes) weekly translations of Chinese-language musings on AI and related topics. Jeff is a PhD candidate in International Relations at the University of Oxford and a researcher at the Center for the Governance of AI at Oxford’s Future of Humanity Institute.
Check out the archive of all past issues here & please subscribe here to support ChinAI under a Guardian/Wikipedia-style tipping model (everyone gets the same content but those who can pay for a subscription will support access for all).
Any suggestions or feedback? Let me know at chinainewsletter@gmail.com or on Twitter at @jjding99