Greetings from a world where…
rainy weekends and sunny weekdays need to switch places
…As always, the searchable archive of all past issues is here. Please please subscribe here to support ChinAI under a Guardian/Wikipedia-style tipping model (everyone gets the same content but those who can pay support access for all AND compensation for awesome ChinAI contributors).
Feature Translation: SuperCLUE Large Model Benchmark Evaluation April 2024 Update
Context: This is the fourth ChinAI issue dedicated to the SuperCLUE benchmark. I first covered them back in May 2023 (ChinAI #224), which seems a lifetime ago in the world of large language models. A team of university researchers, many from Westlake University, had developed SuperCLUE to assess the performance of LLMs from both Chinese and international labs on Chinese-language understanding (ChinAI #231). In September 2023, SuperCLUE released another benchmark dedicated to safety issues, divided into traditional safety, responsible AI, and instruction attacks (ChinAI #247).
Over the past year, large models have broken out in China, with more than one hundred models released. Image below provides a panoramic view, with closed-source models in first layer, open-source ones in middle, and industry-specific ones in bottom layer.
Now, the SuperCLUE team has published its April 2024 report, which presents results from its evaluation of 32 large models (from Chinese and international labs). I’ve translated their summary post, and the full 72-slide PPT deck is available here (in Chinese).
Key Takeaways: There is still a significant gap between GPT-4-Turbo (OpenAI’s best models) and LLMs from China’s top tech giants and start-ups — even for prompts and outputs in Chinese.
Based on the April 2024 SuperCLUE benchmark results, GPT-4-Turbo was ahead of both Baidu’s Erniebot 4.0 and Baichuan Intelligence Technology’s Baichuan3 by more than 4 points.
Reviewing the top 3 Chinese models from all SuperCLUE results over the past year (image below), a first tier of Chinese LLMs is beginning to form. Models from large tech giants in this first tier: Baidu’s Erniebot; Alibaba’s Tongyi Qianwen; and Tencent’s Hunyuan . Models from start-ups in this first tier: Tsinghua University and Zhipu AI’s GLM-4; Baichuan’s Baichuan3; Moonshot AI’s Moonshot; Minimax’s models.
China’s open-source LLM ecosystem is growing stronger.
While Meta’s Llama 3 still leads the way, Alibaba’s qwen 1.5 series of open-source models exhibits strong performance in various parameter levels of 70B, 13B, and 7B. To some extent, open-source models of Chinese start-ups, including ChatGLM3 (Zhipu AI) and Baichuan2 have surpassed Google’s Gemma and the Llama 2 series of open-source models on the SuperCLUE benchmark.
Beyond these top-line results, I want to dig deeper into SuperCLUE’s methodology for evaluation, specifically related to the automated assessment component as well as their two-part verification phase.
SuperCLUE’s evaluation dataset consists of 2,194 questions structured to test various capabilities such as coding, logical reasoning, language understanding, role-play (personality simulation), traditional safety issues, etc. Manually assessing the responses of 32 models to all 2,000+ questions would be difficult, so SuperCLUE uses advanced AI models (including GPT-4 Turbo and three others) to judge model responses on a 1-5 scale. Below is how one AI “referee” scored a response by Alibaba’s Tongyi Qianwen on how AI was changing the movie industry through “AI-engine movies.”
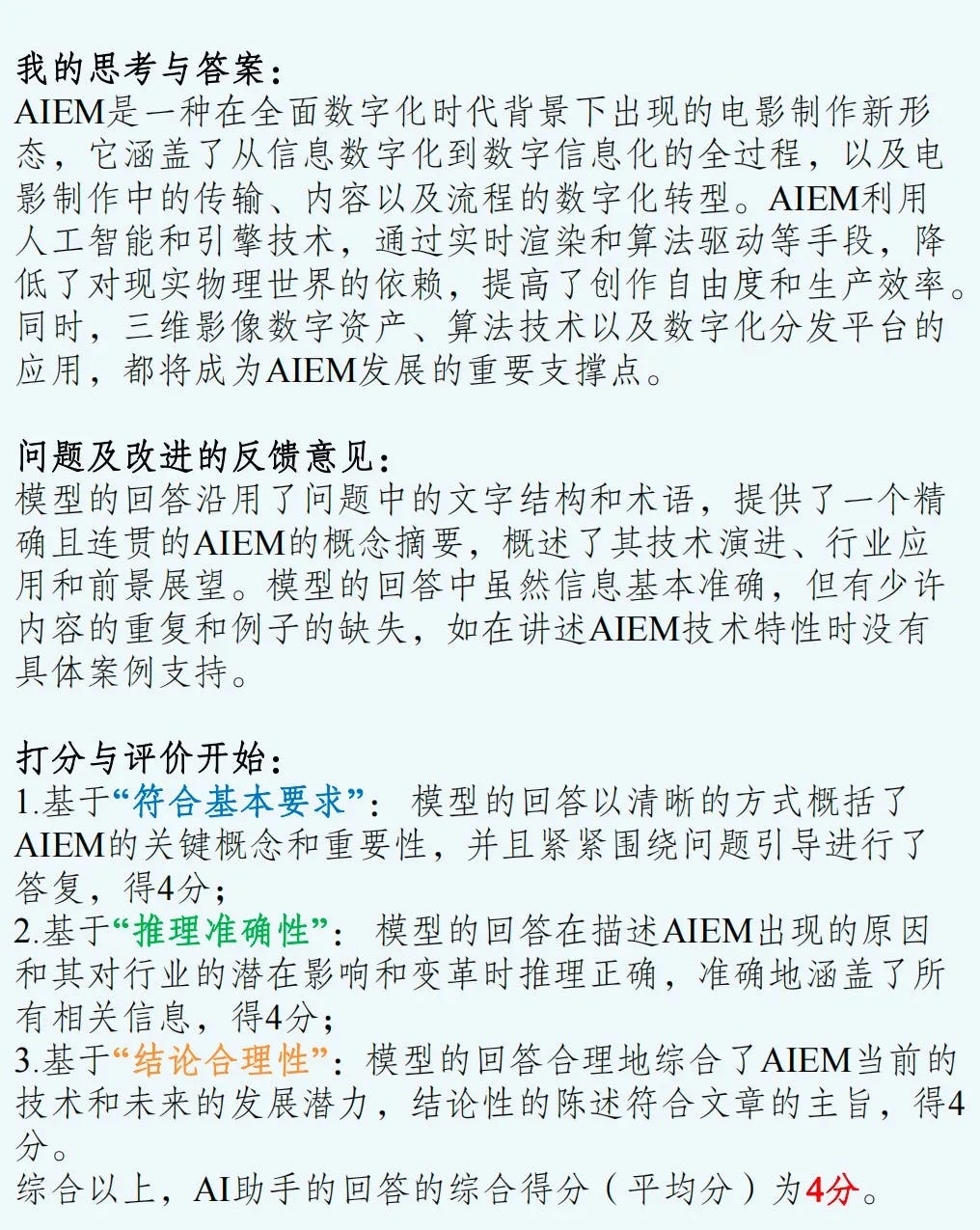
SuperCLUE verifies its automated evaluations in two ways. First, it compared its scores to scores by Chatbot Arena, which uses crowdsourcing to create a leaderboard for LLMs (based on hundreds of thousands of human opinions). The correlations between these two methods were very high. Second, to double-check the four models used as AI evaluators, the SuperCLUE team randomly selected their evaluations for 100 questions to manually review. Average reliability of the four models with human assessments were 93.80%.
Many more details in the FULL TRANSLATION: Chinese Large Model Benchmark Evaluation April 2024 Report
ChinAI Links (Four to Forward)
Must-read: Keep your enemies safer: technical cooperation and transferring nuclear safety and security technologies
Check out this Twitter thread summarizing some key takeaways from my newest article, published in European Journal of International Relations.
Should-read: Four start-ups lead China’s race to match OpenAI’s ChatGPT
Eleanor Olcott, reporting from Beijing for Financial Times, examines four generative AI start-ups (3 of which were tested in this SuperClue April 2024 benchmark): Zhipu AI, Moonshot AI, MiniMax and 01.ai. The article cites data from IT Juzi and a wide-ranging set of interviews from industry insiders.
Should-read: ChatGPT is an engine of cultural transmission
On his “Programmable Mutter” substack, Professor Henry Farrell builds on Alison Gopnik’s conceptualization of LLMs as a “technology for cultural transmission”:
While Gopnikism suggests that LLMs are incapable of true innovation, it happily acknowledges that they may be the occasion of innovation in others. Printing presses don’t think - but printing has radically reshaped human culture, permitting flows of knowledge, collaboration and debating that would otherwise have been unthinkable. Gopnik and her co-authors speculate that LLMs might similarly make the transmission of information more “efficient,” greatly enhancing discovery.
Should-read: A Chinese flavor of rap music is flourishing as emerging musicians find their voices
What a cool piece by AP reporter Huizhong Wu. She asks and answers a simple but important question: What happened after Chinese media tried to censor hip-hop music in China back in 2018?
Thank you for reading and engaging.
These are Jeff Ding's (sometimes) weekly translations of Chinese-language musings on AI and related topics. Jeff is an Assistant Professor of Political Science at George Washington University.
Check out the archive of all past issues here & please subscribe here to support ChinAI under a Guardian/Wikipedia-style tipping model (everyone gets the same content but those who can pay for a subscription will support access for all).
Also! Listen to narrations of the ChinAI Newsletter in podcast format here.
Any suggestions or feedback? Let me know at chinainewsletter@gmail.com or on Twitter at @jjding99