Greetings from a land where yellow peril supports black power…
…as always, the archive of all past issues is here and please please subscribe here to support ChinAI under a Guardian/Wikipedia-style tipping model (everyone gets the same content but those who can pay support access for all AND compensation for awesome ChinAI contributors).
Feature Translation #1: Reactions to GPT-3
Context: xinzhiyuan (AI era), a media portals that focuses on AI, collected some reactions from Chinese netizens to OpenAI’s GPT-3 — the latest language model from OpenAI with 175 billion parameters (GPT-2 was 1.5 billion). The piece takes a lighter approach in terms of reactions, so we’ll unpack some fun memes, but they also feature some interesting quotes from the Zhihu thread on GPT-3.
Key Takeaways:
Zhihu user Li Ru summarized the advantages of GPT-3 over BERT (Google’s language model): BERT's task fine-tuning in specific fields relies too much on labeled data and is easy to overfit, while GPT-3 only requires a small amount of labeled data and no fine-tuning.
Earlier iterations of GPT and GPT-2 lagged BERT in terms of natural language understanding; GPT-3 was better at a few reading comprehension benchmarks but still lagged the fine-tuned BERT/state-of-the-art models in contextual vocab analysis and answering middle school/high school exam questions
Some light-hearted poking fun at OpenAI from netizens: the Zhihu thread (Chinese Quora) on GPT-3 was tagged as 炫富 (wealth-flaunting/show-off-y). Compute used was 2000x that of BERT, and Zhihu netizen “Jsgfery” pointed out how there was a bug in a filtering component of OpenAI’s training process, but due to the cost they couldn’t retrain. In the words of Jsgfery: “The landlord does not have surplus grain to let you train the model again.” (地主家也没有余粮再训练一次了).”
This article was the first time I came across the slang term “调参侠” (“the hyperparameter-tuning knights”) used to refer to ML engineers. The joke is that all AI engineers do is tune hyperparameters, so now that models like GPT-3 are showing that this may not be that necessary, these parameter-tuning knights have nothing to do now.
Some serious stuff in the piece as well: It reflects on how OpenAI did not release GPT-2 and states that “many people agree with the prudent approach of OpenAI.”
***READ FULL TRANSLATION: Reactions to GPT-3
Feature Translation #2: Breakdown of the ACL 2020 Accepted Papers
Context: Another xinahiyuan (AI New Era) piece on some stats and trends from the papers accepted to ACL 2020 — The Association for Computational Linguistics conference, which is a premier venue for publishing NLP research.
Key Takeaways:
Growth of this subfield in recent years is just remarkable: This year’s 3429 paper submissions was an increase of 523 over the previous year; overall, the number of paper submissions more than doubled in the past two years.
Authors at Chinese institutions submitted the most papers, and had the second most accepted papers, but their acceptance rate was outside top 10 countries; US had most accepted papers and a very high acceptance rate. Stats for top three countries by accepted papers follows:
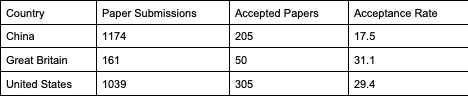
This is from the original article’s breakdown of author affiliations by country (all countries in the original. Some caveats — methodology wasn’t that clear, so I assume they scraped this and didn’t manually identify all authors so there could be some discrepancies through scraping.
Last half of article has summary of ACL 2010-2020 research trends by Professor Che Wanxiang (at Harbin Institute of Tech):
Stark rise in publications on human-machine dialogue starting in 2016 (virtual assistants eating the Internet)
Other subjects that have risen in popularity: new tasks and resources for challenging AI systems, Q&A systems, and text generation
Very surprising to me: ACL publications on machine translation have actually declined since 2013; Che’s explanation is that all these publications have been taken over by Transformer models which can be applied to a variety of NLP tasks (including translation) and can often outperform neural machine translation models in specific tasks.
***READ FULL(ish) TRANSLATION: ACL Breakdown *** I mostly threw up the images from the slides and ACL stats and tried to add some translations/annotations. Just comment in the Google doc if you have questions about anything in particular.
ChinAI Links (Four to Forward)
Must-read: Overcoming Barriers to Cross-cultural Cooperation in AI Ethics and Governance
A group of researchers from the Cambridge Centre for the Study of Existential Risk and the Beijing Academy of Artificial Intelligence published a paper on cross-cultural cooperation in AI ethics and governance in Philosophy and Technology.
In the paper they argue that international cooperation will be essential for ensuring the global benefits of AI, discuss some of the barriers to such cooperation and how they might be overcome. They particularly emphasize the important role that academia may play in cross-cultural cooperation, since academics can often have conversations or engage in collaborations that are difficult in government or industry, and make a number of practical recommendations for things academics can do, including translating key papers and research exchanges. I think it’s a really nice counterbalance to increasing number of articles and reports framing these issues adversarially (especially with respect US-China tech competition).
The full paper is here, and a short blog post describing the key points is available here.
Should-read: AI Definitions Affect Policymaking
How many think tanks in the world have the capabilities to do this: “CSET developed a functional AI definition using SciBERT—a recent neural network-based technique for natural language processing trained on scientific literature (p. 14).” The problem they try to solve: How you define “AI” will significantly affect any claims you make about AI governance, politics, and policymaking. I’ve called this the AI abstraction problem in previous writing.
Should-read: The Innovation Gap by NESTA (2006)
A blast from the past but still relevant, especially to the current US nat sec community’s obsession with achieving tech dominance over China, which I have tastefully analogized to a glorified dick-measuring contest. NESTA convened some of the smartest thinkers on S&T policy (including many from the University of Sussex Policy Research Unit, which is just an incredible hub for brilliant thinkers on this), and tried to develop a more comprehensive innovation strategy for the UK. Coolest part here is that they unpack how traditional innovation indicators (patents, scientific papers) miss “hidden innovation” which doesn’t show up in these statistics. Does anybody know if the U.S. has undertaken a similar effort? Folks beyond my pay-grade should seriously consider just replicating this for the US context.
Should-read: Blitzkrieg, the Revolution in Military Affairs and Defense Intellectuals
Rolf Hobson for Journal of Strategic Studies in 2010:
“It is hard to explain why the defense intellectual milieu has received so little academic attention when it plays so obvious a role in forming American strategic culture and exerts an undeniable influence over both foreign and domestic policy. It must represent one of the most powerful, unelected groups within the American polity, and the purposes served by its research should presumably be the subject of public interest and scrutiny. In any other field, it is assumed – or suspected – that the products of research institutions are influenced by funding, political affiliations, institutional rivalry and individual career structures. In this one, however, their impact on theory can only be guessed at. One insider has compared the quick succession of High Concepts in American strategic debate to the workings of the fashion industry. If that is a valid comparison, it is also justified to ask what mechanisms exist within the industry to weed out the bad theory that fads and market forces will inevitably produce.”
The U.S. needs more of these mechanisms. Gatekeep the gatekeepers. Be very critical and skeptical of “High Concepts:” e.g., Decoupling!, New Tech Cold War, “China Reckoning,” etc.
Thank you for reading and engaging.
These are Jeff Ding's (sometimes) weekly translations of Chinese-language musings on AI and related topics. Jeff is a PhD candidate in International Relations at the University of Oxford and a researcher at the Center for the Governance of AI at Oxford’s Future of Humanity Institute.
Check out the archive of all past issues here & please subscribe here to support ChinAI under a Guardian/Wikipedia-style tipping model (everyone gets the same content but those who can pay for a subscription will support access for all).
Any suggestions or feedback? Let me know at chinainewsletter@gmail.com or on Twitter at @jjding99
6.14.20 NOTE: This post was edited to adjust 1) a typo in GPT-3’s number of parameters, 2) the translated article’s title, and 3) a distinction between hyperparameters vs. parameters.