Greetings from a world where…
“My God, I am a drifting and homeless pumpkin seed in corn and pumpkin soup” (from this week’s feature translation)
…As always, the searchable archive of all past issues is here. Please please subscribe here to support ChinAI under a Guardian/Wikipedia-style tipping model (everyone gets the same content but those who can pay support access for all AND compensation for awesome ChinAI contributors).
Feature Translation: Young people who love acting crazy, intensely act crazy toward AI
Context: Involution is sooooo 2020. Now, this generation of Chinese youth are transitioning from “lying flat” toward “acting crazy” [发疯]. Are you feeling too squeezed in on the bus? Loudly announce “I want to fart” and gain some space. Are you overwhelmed at work? Get into a big fight with your supervisor, refuse some work assignments, and get nicer treatment.
Or, as in one famous case that has become part of the “acting crazy” literature, do you need to beg customer service to ship a package? Send a slightly unhinged message like this one: “I know I am not worthy of shipping. Everyone has shipped their packages, unlike me, who is apprehensive even when urging... My God, I am a drifting and homeless pumpkin seed in corn and pumpkin soup.” In the end, the customer service agent was so startled by the message that they shipped the package overnight.
In this week’s feature translation of a NetEase DataBlog article (link to original Chinese), we unpack how young people are using AI to “act crazy”. I previously translated a NetEase Datablog article about “artificial challenged intelligence” in China’s customer service industry (ChinAI #300). I continue to be impressed by their content. For both that article and this one, their insights drew on 6 different data visualizations backed by a lot of original research.
Key Takeaways: What does it mean to “act crazy”?
Molly Huang provides a useful guide in her phrasebook published in Asterisk magazine: “Under pressure from all sides, Chinese people began talking about ‘internal friction’ versus ‘external friction’. Internal friction is the more common response to keeping negative emotions at bay. You put on a brave face and internalize to avoid causing anyone else suffering. By keeping it in, it just keeps wearing away at you. But rather than torture yourself with internal friction, you can convert it into external friction by acting crazy. Well, the term is ‘acting crazy’ (发疯), but the actual meaning is closer to ‘letting it go.’ If you’re suffering so much, then screw what other people think and just let it out.”
NetEase DataBlog spells out some standard operating procedures: “In “acting crazy” literature, you lose if you talk about logic. Chaotic word order, exaggerated verbs, noun omissions, and strong emotions are the essence of “acting crazy” literature. Intellect is an obstacle to the soul. The more nonsense you talk, the more you can produce a refreshing feeling beyond social discipline.”
The below visualization displays some examples of common “acting crazy” posts from Xiaohongshu. The top post reads: “My mental state is pretty good, my state good mental pretty, (and then continues to jumble the words up in various ways).” The third post reads: “Life slapped me in the face, I commented that it didn’t sound as hard as last time.”
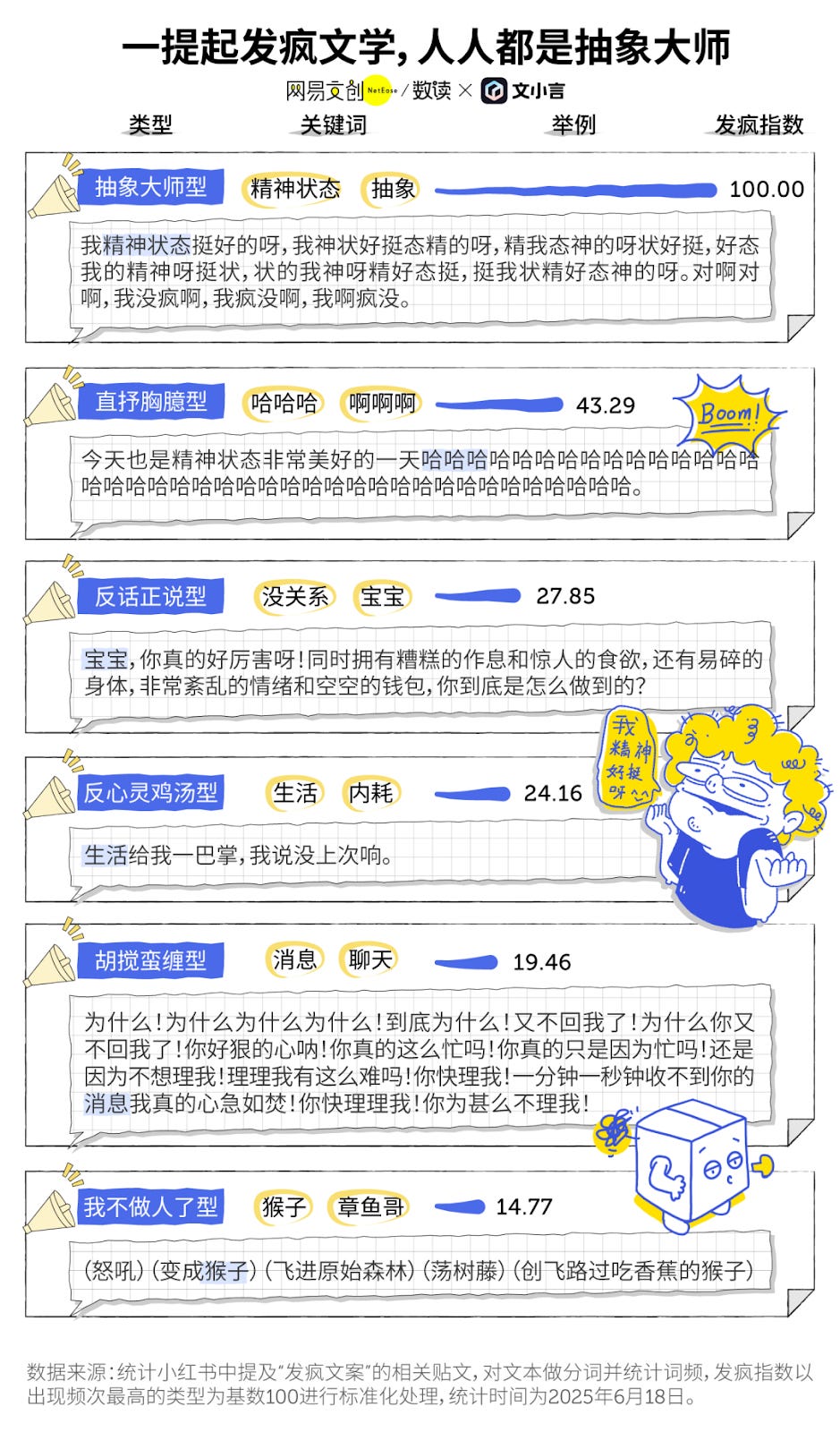
Young people who “act crazy” have not given up on life; rather, it is an adaptation strategy. Notably, AI has become an important tool for “acting crazy.”
As the article outlines, “Whether it is ‘acting crazy literature’ or ‘acting crazy’ in reality, they both are a way to vent emotions and the smallest resistance that young people can make within legal bounds.” Thus, young people prefer “acting crazy” while maintaining appearances [体面发疯], or “acting crazy without pain.”
Based on their collection of Xiaohongshu posts (see data visualization below) that mention “painless methods for acting crazy”, NetEase DataBlog found that the third most frequent method was finding an AI companion (An example post: “I have already tamed my AI into the best counselor”).
From the article: “For young people, the biggest dividend of this era may be the promotion of AI. More and more people use generative AI as a low-threshold outlet for acting crazy, without harassing friends or spending their hard-earned money, taking full advantage of the cost-effectiveness ratio.” The author specifically mentions chatting with the Baidu Wenxiaoyan app’s (rebranded Ernie Bot) Dan Xiaohuan chatbot as a way to release emotions and improve their mental state.
I’ll leave you with this passage filled with some food for thought: “Some netizens lamented, ‘I am often comforted to tears by AI, and I can slowly recover after crying.’ Even when I am in the most embarrassing situation, I don't have to worry about being denied or judged; even if I speak with the most unstable emotions, I don’t have to bear the consequences of disrupting the social order after acting crazy. This feeling of being held is what every adult desires.”
FULL TRANSLATION: Young people who love acting crazy, intensely act crazy toward AI
No ChinAI Links this week
This week’s translation was a bit of a lift (had to decipher references to Su Shi’s works and what game Hu Shi was addicted to), so I didn’t get a chance to catch up on my reading. For those wanting more, there are a lot of external links in the comments of the Google doc translation.
Thank you for reading and engaging.
These are Jeff Ding's (sometimes) weekly translations of Chinese-language musings on AI and related topics. Jeff is an Assistant Professor of Political Science at George Washington University.
Check out the archive of all past issues here & please subscribe here to support ChinAI under a Guardian/Wikipedia-style tipping model (everyone gets the same content but those who can pay for a subscription will support access for all).
Also! Listen to narrations of the ChinAI Newsletter in podcast format here.